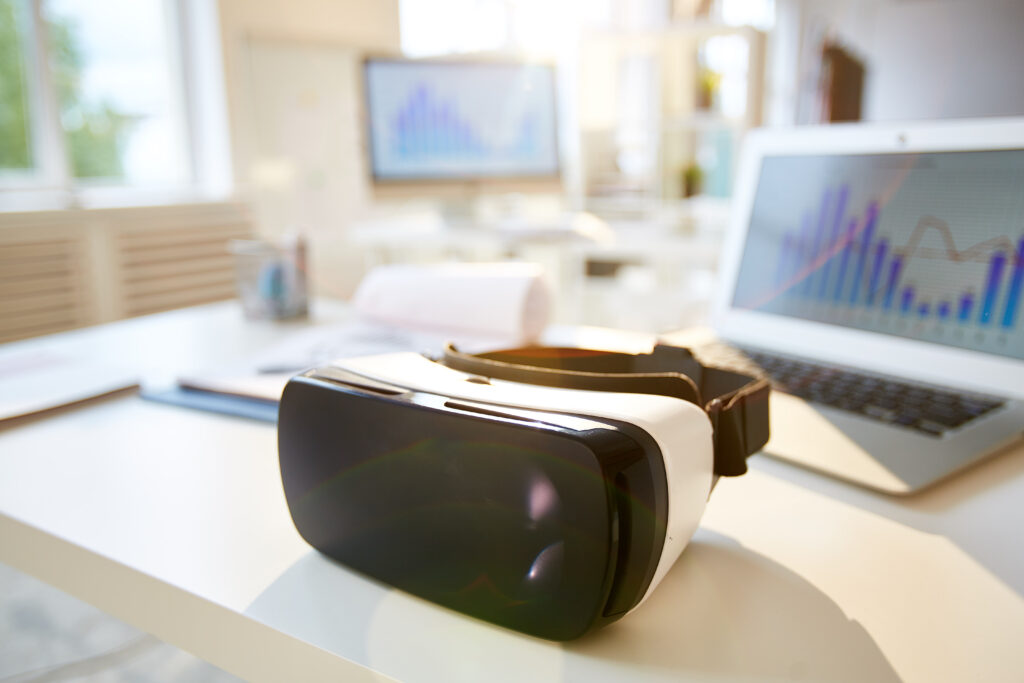
More than just a mathematical tool, Monte Carlo simulations represent the power of chance in making sense of the world’s uncertainties. These probabilistic simulations continue to shape how businesses, scientists and engineers deal with high uncertainty. Going forward, this method will only grow more powerful, offering new ways to predict, optimise, and improve outcomes in a world that is increasingly defined by uncertainty.
Imagine walking into the iconic Monte Carlo Casino where the roulette wheel spins, the dice roll, the machines scream, and fortune hangs in the balance at every moment. The outcome is unknown, shaped by chance and uncertainty – that thrill underpinning the essence of what makes the casino so captivating. However, it is also this very unpredictability that inspired a mathematical breakthrough, transforming the essence of gambling into a powerful tool for understanding complex systems. The casino’s name would become forever linked to a mathematical technique designed to tackle uncertainty – not in gambling, but in predicting complex real-world systems.
This technique, known as a Monte Carlo simulation, uses random sampling to model uncertainty and predict the likelihood of various outcomes. Much like a gambler relies on the unpredictability of the roll of a dice, Monte Carlo simulations embrace randomness to model everything from financial markets to particle physics. But how exactly did this method evolve from a game of chance to one of the most powerful tools in modern science and business?
How Monte Carlo Simulations Work
Monte Carlo simulations take their name from the famous casino in Monaco, renowned for its games of chance and uncertainty. The method mimics this randomness, using it to estimate solutions for complex problems that are difficult to solve analytically. The technique was developed during World War II by mathematicians Stanislaw Ulam and John von Neumann as part of the United States’ nuclear weapons research under the Manhattan Project.
Ulam, who was researching neutron behaviour in nuclear reactors, realised that instead of exact calculations, probabilistic simulations could yield useful insights into the system’s behaviour. Von Neumann refined this approach, creating a formal method that utilised random sampling to approximate solutions in complex situations. The breakthrough was revolutionary, enabling scientists and engineers to tackle problems previously thought unsolvable with traditional deterministic methods.
The basic concept behind Monte Carlo simulations is deceptively simple: simulate a system multiple times using random inputs and observe the variety of outcomes. In practice, it involves four key steps:
- Define a domain of possible inputs: Identify the variables that influence the model and determine their probability distributions – such as normal, uniform, or Poisson distributions – that define the range of possible values.
- Generate random inputs: Randomly sample values for each input variable according to the specified distributions.
- Simulate the model: Run the model using the generated random inputs, calculating an output for each iteration.
- Repeat the process: The process is repeated thousands, if not millions, of times to build a distribution of possible outcomes. The results are then statistically analysed to estimate probabilities and assess risk.
Monte Carlo simulations are particularly useful when exact solutions are impossible or infeasible due to the complexity of the system or the uncertainty surrounding its inputs.
Turning Randomness into Insight
Monte Carlo simulations are used in a variety of industries to model complex systems and make informed decisions under uncertainty. In the financial world, for example, investment banks like Goldman Sachs extensively use Monte Carlo methods for risk management and portfolio optimisation. Analysts simulate thousands of possible scenarios to assess the risk of an investment portfolio, modelling fluctuations in asset prices, interest rates and market conditions and expectations. The method is also widely used in derivatives pricing, where options and futures are valued based on market randomness.
In engineering, these simulations are employed to predict the reliability of systems and components under various conditions. Engineers can optimise designs and assess the likelihood of system failure by running simulations on manufacturing processes or machinery. This is crucial in fields like aerospace, automotive design and even software engineering, where reliability and performance are paramount.
NASA, for example, models the behaviour of spacecraft during various stages of a mission using Monte Carlo simulations. Given the unpredictable nature of space environments, these help predict outcomes such as spacecraft failure, fuel consumption or trajectory adjustments. This allows engineers to plan for a variety of potential scenarios and minimise the risk of mission failure.
Automotive and energy companies like Tesla too model complex systems, from vehicle performance under various driving conditions to the lifespan of energy storage systems, like batteries, helping optimise designs and improve efficiency, reliability, and safety.
The healthcare sector also benefits, especially in clinical trials and epidemiological studies. Researchers use the method to simulate the effects of different treatments or interventions, as well as to predict the spread of diseases in populations. This enables healthcare providers to better allocate resources and make decisions that minimise risk while maximising benefit.
Leading tech companies in the world of artificial intelligence (AI) and machine learning like Google and Microsoft also use Monte Carlo simulations in reinforcement learning, where an agent interacts with an environment and learns to make decisions based on simulated scenarios. The method helps AI models explore various strategies by simulating outcomes in uncertain environments, allowing the system to optimise its performance over time. Google’s DeepMind for example uses Monte Carlo Tree Search (MCTS) algorithms to solve complex problems, from playing board games like Go to navigating robot decision-making processes
While Monte Carlo simulations are incredibly powerful, they are not without challenges. One of the primary obstacles is the computational complexity of the method. Simulating large systems with many variables requires running millions of iterations, which can be time-consuming and computationally expensive. As the size and complexity of the model grow, so too does the computational cost.
Another challenge is the accuracy of input distributions. The effectiveness of Monte Carlo simulations depends heavily on the assumptions made about the probability distributions of input variables. If these distributions are inaccurate or poorly chosen, the simulation’s results can be misleading, which can be particularly problematic in fields like finance and healthcare, where decisions based on incorrect models can have significant real-world consequences.
Moreover, convergence issues can arise when the simulations do not produce stable results after a large number of iterations. This is particularly true for models with high-dimensional input spaces, where random sampling might not adequately cover the entire range of possibilities. As a result, Monte Carlo simulations may fail to capture rare but important events, especially in extreme cases or “tail” distributions.
Looking ahead, Monte Carlo simulations are poised to benefit from significant advancements in computing power. The emergence of quantum computing holds the promise of exponentially speeding up simulations by leveraging quantum bits (qubits) to explore many possibilities simultaneously. This could revolutionise Monte Carlo simulations, particularly in fields like cryptography, financial modelling, and material science.
Additionally, the integration of machine learning techniques with Monte Carlo simulations is likely to enhance their efficiency and accuracy. AI-driven optimisation algorithms could help identify the most critical input parameters and optimise the number of required simulations, reducing computational costs and improving convergence.
As industries continue to tackle increasingly complex and uncertain problems, Monte Carlo simulations will remain a critical tool in their decision-making arsenals. From optimising energy systems to guiding autonomous vehicles, the method’s future is bright, with new applications and innovations on the horizon.