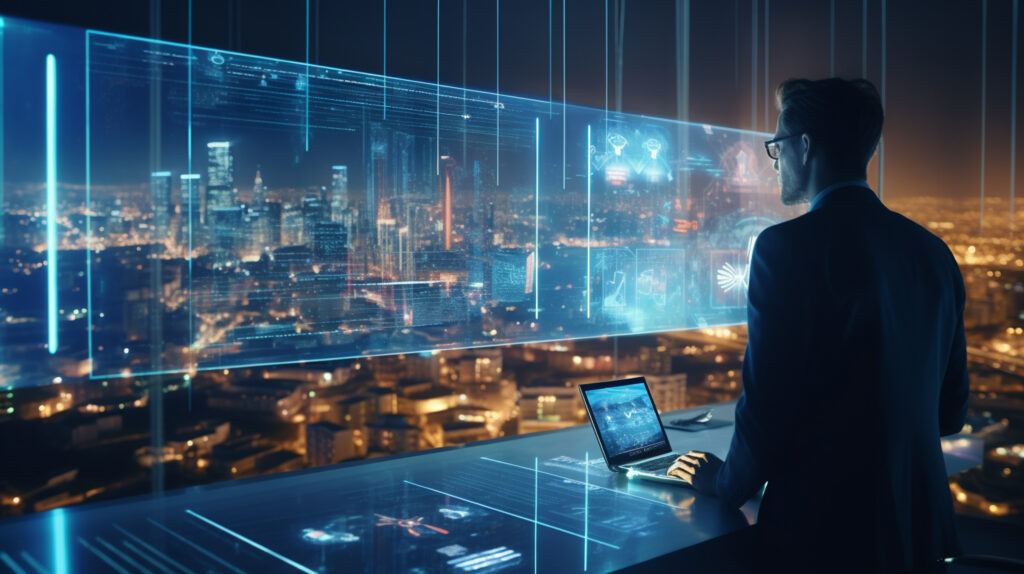
Scaling AI is not merely about deploying more algorithms; it’s about building a robust infrastructure that supports sustainable growth. From data pipelines and compute power to governance frameworks, the journey to enterprise-scale AI demands vision, investment, and adaptability. Those who build the right foundations today will not only lead the AI revolution but redefine what’s possible in the digital economy.
Despite Artificial Intelligence (AI) moving from a buzzword to a critical driver of business innovation, promising efficiency, personalisation, and transformative insights, scaling it within enterprises remains a daunting task, as organisations grapple with technical, infrastructural, and strategic challenges. While AI pilots often succeed in isolation, scaling them enterprise-wide demands a robust and integrated infrastructure – a necessity that separates market leaders from laggards.
The urgency to scale AI is underscored by a surge in demand for automation, hyper-personalisation, and real-time insights. According to McKinsey’s 2024 AI adoption report, only 11% of organisations report achieving significant financial impact from AI – largely because of scaling barriers. Meanwhile, regulatory landscapes such as the EU’s AI Act are forcing companies to rethink their AI infrastructure to ensure transparency and compliance. These pressures make enterprise-scale AI not just a competitive advantage but a business imperative.
The Three Pillars of Scaling AI Infrastructure
Scaling AI at the enterprise level demands an architecture that can manage complexity, enable adaptability, and ensure ethical deployment. The foundational pillars of infrastructure include:
- Data as the Lifeblood:AI systems thrive on data, and at scale, data management becomes both an opportunity and a challenge. Enterprises must establish robust pipelines that handle vast, heterogeneous datasets seamlessly.
- Data Engineering Pipelines: Automating data ingestion, cleaning, and transformation to ensure models have consistent and high-quality inputs.
- Data Lakes and Warehouses: Platforms like Snowflake and Databricks that provide centralised, scalable data storage while supporting analytics in real time.
- Federated Learning: An emerging approach where data remains decentralised, allowing enterprises to train AI models without compromising privacy.
Retail giant Walmart demonstrates how centralised data management drives scalable AI. Using an advanced data lake, Walmart integrates customer data from its e-commerce platform, in-store sales, and supply chain to generate actionable insights. These insights power inventory predictions, personalised promotions, and dynamic pricing at scale.
- Compute Infrastructure for Scalability:AI models are computationally intensive, requiring infrastructure that balances speed, scalability, and cost-effectiveness.
- Edge Computing: To reduce latency, organisations are moving computations closer to data sources, especially in IoT-heavy industries.
- Hybrid Cloud Solutions: Platforms like AWS, Google Cloud, and Azure offer the scalability of public cloud with the security of on-premises systems.
- GPU and TPU Clusters: High-performance processing units are essential for training complex models like GPT-4 or image recognition systems.
Tesla’s Dojo supercomputer illustrates the importance of cutting-edge computer infrastructure. By deploying a custom-built system optimised for neural network training, Tesla achieves faster processing for its autonomous driving AI, giving it a technological edge in the competitive EV market.
- Operationalising AI through MLOps:Machine Learning Operations (MLOps) integrates development (DevOps) and data science workflows to ensure AI systems are robust, reliable, and adaptable.
- Model Lifecycle Management: Continuous monitoring and retraining to address data drift and maintain performance.
- Deployment Pipelines: Automating the deployment of models across environments, reducing time-to-market.
- Governance Frameworks: Tools that ensure model explainability, fairness, and compliance with regulations.
Healthcare company Anthem, now Elevance Health, uses MLOps to scale AI solutions for predictive analytics. By automating workflows, Anthem significantly reduced errors in claims processing and improved patient outcomes through targeted care recommendations.
Despite its promise, enterprise-scale AI faces several roadblocks:
- Talent Shortages: AI initiatives often stall due to a lack of skilled professionals who understand both technical and business contexts. Companies must invest in upskilling teams or leveraging external partnerships.
- Ethical and Regulatory Complexities: AI systems at scale amplify ethical concerns, from bias in decision-making to data privacy violations. Building frameworks for ethical AI deployment is non-negotiable.
- Cost Constraints: Scaling AI requires significant upfront investment in technology and infrastructure, often deterring smaller organisations. Cloud-first strategies and managed AI services can alleviate some of these costs.
To overcome the challenges of scaling AI, enterprises must align technology with their strategic vision.
Success begins with high-impact use cases that deliver measurable ROI, such as fraud detection, supply chain optimisation, or customer segmentation, fostering momentum for broader adoption. Cross-functional teams combining data scientists, engineers and business leaders are essential to bridging technical and operational gaps. Partnerships with cloud providers, AI startups and academic institutions can provide access to cutting-edge tools and expertise, while robust governance – through AI ethics boards and audit mechanisms – ensures compliance and builds stakeholder trust.
Looking ahead, the scalability of enterprise AI hinges on addressing today’s challenges. Emerging technologies like quantum computing and synthetic data are poised to revolutionise AI infrastructure, making it more powerful and accessible. But the race is not solely technological – it is also strategic. Companies that treat AI as a long-term investment rather than a one-off project will define the benchmarks for innovation and operational excellence in the years to come.forts.