Scaling GenAI efficiently and cost-effectively is all about balance between performance and cost, flexibility and control. Through leveraging Cloud infrastructure, optimising model training, using specialised hardware, and focusing on governance, businesses can harness the power of AI without overextending their budgets.
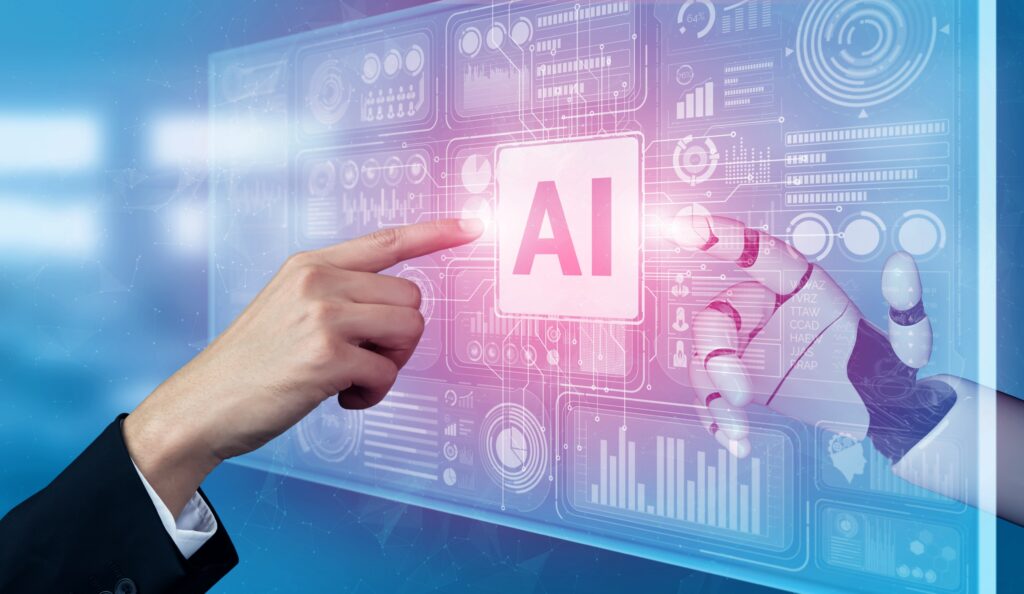
Generative AI is quickly becoming a vital tool for businesses across industries, powering everything from content creation to predictive analytics. Its potential to generate new ideas, automate complex tasks, and offer fresh insights is transforming how companies operate. However, as powerful as Generative AI is, it also comes with a challenge: deploying and scaling these models in a way that’s efficient and cost-effective.
For many organisations, the steep computational and financial demands of Generative AI can seem daunting. But with careful planning, the right infrastructure, and smart strategies, businesses can unlock the benefits of this technology without breaking the bank.
The Infrastructure Demands of Generative AI
Generative AI models, such as GPT or image generation systems like Stable Diffusion, are built on massive neural networks that require significant computational resources. Training these models involves handling vast datasets and performing complex calculations, which can be both time-consuming and costly.
For most businesses, building the necessary in-house infrastructure is neither practical nor affordable. This is where Cloud computing comes in. Cloud providers like AWS, Google Cloud, and Microsoft Azure offer access to powerful computing resources on a pay-as-you-go basis, allowing companies to scale up or down as needed. By leveraging the Cloud, businesses avoid the upfront costs of purchasing and maintaining hardware while still having access to high-performance infrastructure.
The key is to choose a Cloud service that matches the specific needs of AI projects. Optimised instances for AI workloads, for example, ensure that businesses only pay for the computational power they actually need, avoiding waste.
For businesses that already have some on-premises infrastructure, or those that want to ensure greater flexibility, hybrid Cloud solutions offer a compelling option. A hybrid approach combines on-premises systems with Cloud-based resources, allowing companies to scale up when needed, but without fully committing to one environment.
Similarly, multi-Cloud strategies, where businesses utilise services from multiple Cloud providers, offer an additional layer of flexibility. By spreading AI workloads across different Clouds, organisations can optimise for performance, availability, and cost. For example, heavy computational tasks might be run on a Cloud provider that specialises in high-performance GPU instances, while less intensive processes can be handled by a more cost-effective platform. This approach helps businesses stay agile while keeping expenses manageable.
Training Generative AI models is where the bulk of costs are incurred. However, several techniques can help reduce both the time and expense involved:
- Transfer Learning: Instead of building models from scratch, transfer learning allows businesses to start with pre-trained models and fine-tune them for specific tasks. This drastically cuts down the computational power required and speeds up the process.
- Batch Processing: Processing data in batches during model training reduces the number of iterations, making the training process more efficient.
- Model Pruning and Quantization:These techniques reduce the size and complexity of AI models by trimming unnecessary parameters, allowing them to run faster without losing accuracy.
- Spot Instances: Many Cloud providers offer discounted “spot instances” when spare capacity is available. These can be used for non-urgent AI tasks, offering significant savings compared to standard pricing.
Deploying AI Models for Scalability
Once the AI model is trained, the next challenge is deployment. To deploy AI models efficiently and at scale, businesses need to focus on flexibility and resource management.
One effective approach is using containerisation, where AI models are packaged with all their necessary dependencies. Containers can then be deployed across different environments, ensuring that the model runs consistently whether it’s on a local server, in the Cloud, or in a hybrid setup. Platforms like Kubernetes make it easy to orchestrate and scale containerised applications, automatically adjusting resources based on real-time demand. This ensures businesses aren’t paying for idle infrastructure during downtime.
It is, however, crucial to note AI models thrive on processing power, and not all hardware is created equal. GPUs (Graphics Processing Units) have long been the standard for accelerating AI workloads, but newer specialised hardware, such as TPUs (Tensor Processing Units) and NPUs (Neural Processing Units), is pushing efficiency to new levels.
Cloud providers now offer on-demand access to this specialised hardware, so businesses don’t need to invest in expensive, purpose-built machines. For example, NVIDIA-powered GPUs available on platforms like AWS are designed to accelerate deep learning tasks, making it easier to train and deploy models in less time and with fewer resources.
Monitoring and Managing AI for Long-Term Success
Even when the AI model is live, the work isn’t over. Continuous monitoring is essential to ensure the model is performing as expected and to avoid unnecessary resource usage. AI models, especially generative ones, can become outdated as the data they were trained on evolves. Regular monitoring helps identify when retraining is needed, preventing performance degradation.
Additionally, monitoring tools can help identify inefficiencies in the infrastructure supporting the AI model. By tracking resource usage, companies can spot areas where too much computing power is being consumed, allowing them to optimise and cut down on costs.
It goes without saying – with great AI power comes great responsibility. Governance and compliance play a critical role in ensuring that AI systems are not only effective but ethical and legally sound. Data privacy laws such as GDPR must be adhered to, and businesses should implement governance frameworks to manage the ethical implications of AI.
Many Cloud providers offer tools to help ensure compliance, but regular audits and reviews should also be conducted to make sure AI models are operating within legal and ethical boundaries. By focusing on governance, businesses avoid costly regulatory missteps and build trust with customers. The future of AI holds immense promise, and with the right approach, businesses can unlock its full potential in a way that’s both innovative and sustainable.