The NVLM-D-72B, using 72 billion parameters, is great at understanding both images and text. Its impact will unfold over time, possibly leading to unprecedented collaboration and innovation in AI while also necessitating responsible use.
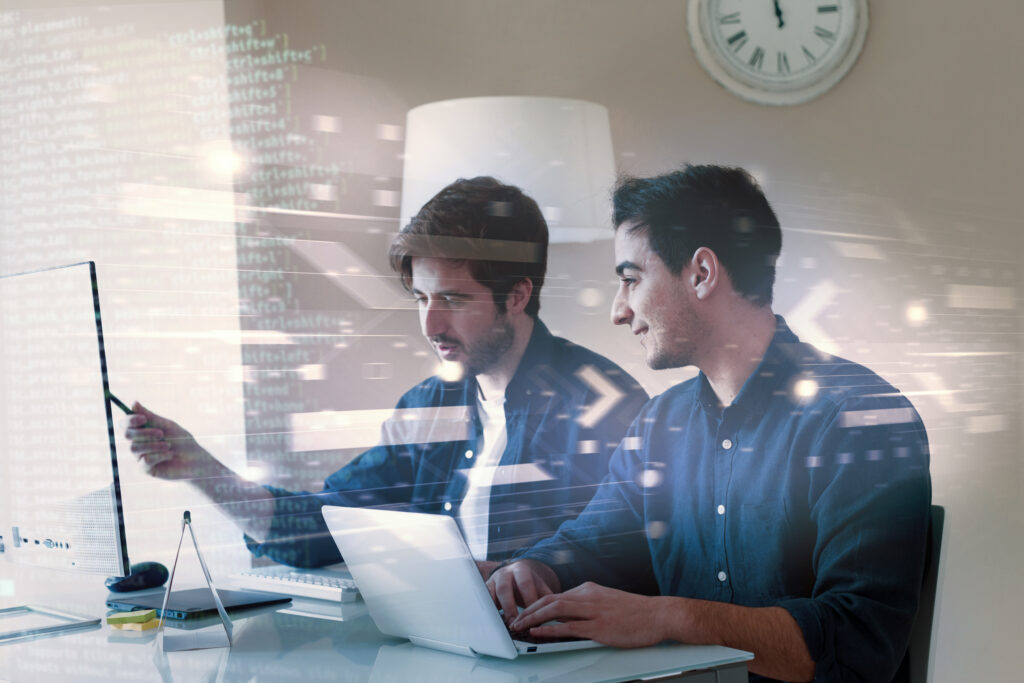
Nvidia has fired a shot across the bow of the entrenched LLM (large language model) players like OpenAI, Perplexity or Anthropic. Its NVLM-D-72B, using 72 billion parameters is great at understanding both images and text, making it versatile for tasks like interpreting memes or solving math problems visually. Imagine a master chef who can create a multi-course meal that combines flavours from around the world. This chef not only excels at cooking but can also beautifully present dishes and even explain the recipes step-by-step.
The NVLM 1.0 family of large multimodal language models, challenges the trend of closed AI systems, providing unprecedented access to cutting-edge technology for researchers and developers. This move could spark a chain reaction. Other tech leaders may feel pressure to open their research, potentially accelerating AI progress across the board. It also levels the playing field, allowing smaller teams and researchers to innovate with tools once reserved for tech giants.
The NVLM-D-72B demonstrates impressive adaptability in processing complex visual and textual inputs, improving text performance by an average of 4.3 points after multimodal training. The AI community has responded positively, noting its competitive performance compared to models like GPT-4 and Llama 3. Nvidia’s open-source initiative could accelerate AI research, enabling smaller organisations to contribute significantly.
A Pivotal Moment in AI
This release marks a pivotal moment in AI development, potentially prompting other tech leaders to open their research. However, it raises concerns about misuse and ethical implications, as well as questions about future AI business models. The impact of NVLM 1.0 will unfold over time, possibly leading to unprecedented collaboration and innovation in AI while also necessitating responsible use.
Nvidia’s open-source LLMs offer significant advantages in customisation, accessibility, and data privacy, allowing organisations to modify models for specific tasks and run them on-premises, which is ideal for sensitive applications. In contrast, proprietary models like OpenAI’s GPT, Claude, and Perplexity provide user-friendly, ready-to-use solutions via APIs, making them suitable for broad applications without the need for extensive technical expertise.
While Nvidia’s models can be optimised for specific hardware and foster community collaboration, the proprietary models often come with usage-based costs and less control over data, making them more convenient but potentially limiting for organisations with specialised needs.
Gourmet Chef & Efficient Cook
Carrying on with the chef analogy,OpenAI’s GPT-4 is like a gourmet chef who focuses on craftingexquisite dishes with complex flavours. This chef is exceptional at writing and generating text, creating stories, essays, or code. While they can handle some visual tasks, their main strength lies in producing high-quality written content.Perplexity as a quick and efficient cook who specialises in making simple, tasty meals. They’re great at answering questions and providing straightforward information quickly, but they may not have the depth of flavour or creativity found in more specialised chefs. Claude would be a chef known for their ability to whip up comfort food that feels familiar and warm. This chef is reliable and good at understanding what people want, but they might not venture into experimental dishes as much as others.
While all these chefs (AI models) can cook (process information), Nvidia’s NVLM-D-72B stands out because it can blend different cooking styles (text and images) and tackle a broader range of tasks, making it adaptable and innovative in ways that others may not be.
Meets High Levels of Customisation Needs
Nvidia’s open-source LLMs excel in use cases that require high levels of customisation, control, and integration with existing systems. For instance, organisations in specialised fields such as healthcare or finance can fine-tune these models on proprietary datasets, ensuring that the outputs are not only relevant but also compliant with industry regulations. This capability allows for the development of tailored applications like patient record analysis or financial report generation, where data privacy is paramount. Additionally, companies can leverage Nvidia’s models to optimise performance on their specific hardware, resulting in faster inference times and reduced operational costs.
In contrast, while proprietary models like OpenAI’s GPT, Claude, and Perplexity offer ease of use and quick deployment through APIs, they often limit users to predefined functionalities and can incur significant costs with increased usage. For example, a startup might find it more economical and efficient to deploy Nvidia’s models on local servers for customer support chatbots, allowing for greater scalability without escalating expenses. Furthermore, the open-source nature of Nvidia’s LLMs encourages community collaboration, leading to rapid advancements and shared innovations, which can drive further enhancements in performance and capabilities. This combination of flexibility, cost-effectiveness, and community support positions Nvidia’s open-source models as a superior choice for organisations with specific and evolving needs.