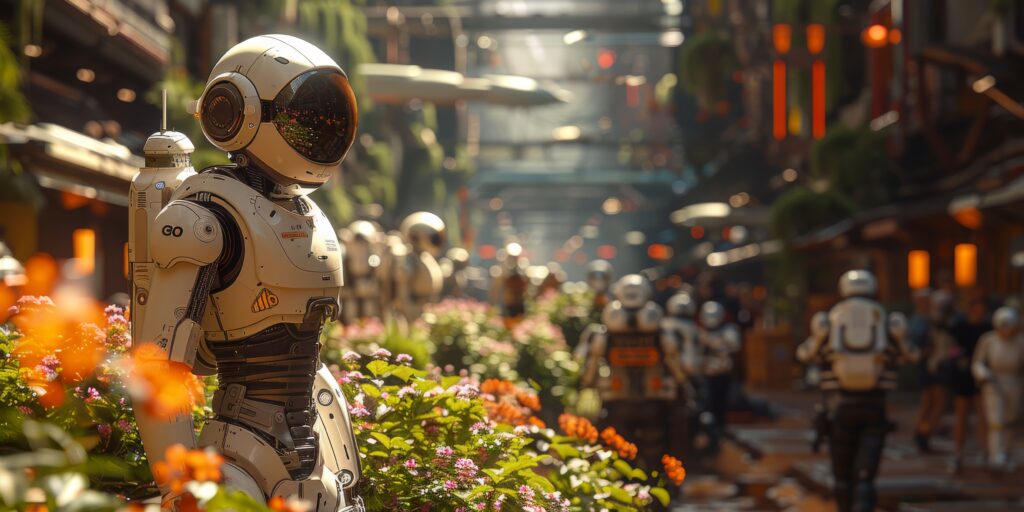
Robotics development has been profoundly affected by Moravec’s Paradox. Rooted in evolutionary biology, it serves as a reminder that the ability to perceive, feel, and interact with the world is a complexity that is still beyond the reach of current roboticists continue to change, so do the ways in which we measure success!
In a world where robots perform the most complex of calculations at lightning speed, the simple act of picking up a pencil still isn’t straightforward. This phenomenon, known as Moravec’s Paradox, is reshaping the development of robotics and artificial intelligence in unexpected ways. It serves as a reminder that what makes us human – the ability to perceive, feel, and interact with the world – is a complexity beyond the reach of current robotics.
Moravec’s Paradox is the observation that tasks humans find difficult, such as high-level reasoning or solving complex problems, are often easier for computers and robots to perform. Conversely, tasks humans perform effortlessly, like walking, grasping objects, or understanding nuanced emotional expressions, are difficult for machines to master. Hans Moravec, along with researchers like Rodney Brooks, pointed out this paradox in the 1980s, observing that the things humans learn in early childhood – including motor skills and sensory perception – are far more complex than advanced mathematical reasoning from a computational standpoint.
The paradox is rooted in evolutionary biology. Human brains evolved to handle sensory input, motor control, and environmental interaction over millions of years, while abstract reasoning and logic are relatively recent developments. As a result, these “easy” tasks are deeply embedded in our neural networks, whereas tasks involving reasoning and computation are not.
Robotics and Moravec’s Paradox
Robotics development has been profoundly affected by Moravec’s Paradox. Early research assumed that high-level reasoning would be the hardest task for robots, but breakthroughs in computing power and artificial intelligence have shown otherwise. Computers can now perform sophisticated data analysis and logical operations at speeds no human could achieve. However, even the most advanced robots still struggle with basic tasks that require interaction with the physical world.
For instance, self-driving cars can process immense amounts of data from their surroundings and make split-second decisions, but they struggle with scenarios that require understanding human intent or performing delicate tasks in unpredictable environments. Similarly, humanoid robots like Boston Dynamics’ Atlas can run, jump, and even backflip, but their ability to interact with objects or people in a meaningful way remains limited.
This limitation can be seen in the healthcare sector, as noted in a Lancet article discussing robotic interventions in medical environments. Robots have demonstrated proficiency in tasks like diagnostics and data analysis, but they are less effective in caregiving roles, which require emotional intelligence, physical dexterity, and the ability to respond to subtle human cues. These gaps illustrate the paradox’s impact on the deployment of robotics across industries that depend on both technical skill and human empathy.
Overcoming Moravec’s Paradox requires advancements in how robots perceive and interact with the world. Current artificial intelligence (AI) technologies rely heavily on large-scale data analysis and pattern recognition, but they still fall short when it comes to mimicking the subtleties of human behaviour. While robots excel in controlled environments with predictable outcomes, they falter in chaotic or dynamic settings.
One solution being explored is the integration of machine learning with real-world interaction. By allowing robots to learn through experience, researchers hope to bridge the gap between cognitive and physical skills. However, this approach presents its own challenges. Machine learning algorithms need vast amounts of data to learn even basic physical tasks; and even then, they often lack the common sense or adaptability of a human child.
For example, teaching a robot to tie a shoelace may require thousands of repetitions, while a child could learn the same task after watching someone else do it a few times. Furthermore, robots struggle with “transfer learning” – the ability to apply knowledge from one domain to a different but related task. This limitation significantly slows the progress of robotics in fields where adaptability is crucial.
Moravec’s Paradox highlights the need for a more nuanced approach to developing robots that can interact with the world in meaningful ways. Robotics research is shifting from focusing solely on abstract intelligence to prioritising sensory and motor skills that mirror human capabilities.
One promising development is the use of embodied cognition, where robots learn by physically interacting with their environment, much like humans do. This approach, coupled with advanced AI models, is helping to develop robots that are better suited for tasks like caregiving, where emotional understanding and physical dexterity are crucial. These advancements could lead to robots capable of performing complex physical and emotional tasks, from providing elderly care to assisting surgeons in delicate operations.
Additionally, the paradox underscores the importance of collaboration between humans and robots. Instead of replacing human labour entirely, the future of robotics may lie in augmenting human abilities. Robots could handle the complex, high-volume data processing tasks that humans find difficult, while humans focus on tasks that require emotional intelligence, creativity, and dexterity.
While robots will continue to advance in intelligence and capability, their development is likely to remain constrained by their limited ability to perform simple, everyday tasks. As researchers work to bridge the gap between robotic and human abilities, the paradox will continue to shape the evolution of robotics, guiding the next wave of technological innovation in surprising ways.