Beyond Algorithmic Bias
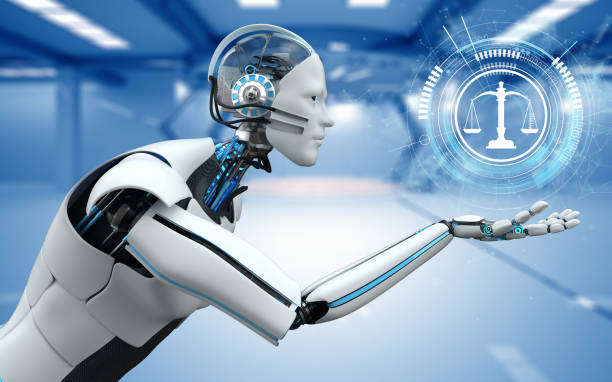
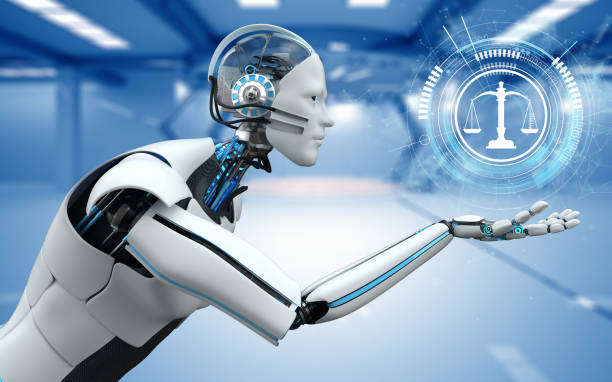
To prevent detrimental feedback loops and promote equitable AI adoption, it is crucial for stakeholders to collaborate and prioritise human-centric and equitable outcomes
Artificial Intelligence (AI) and machine learning are rapidly transforming our world, promising breakthroughs in healthcare, automation of mundane tasks, and substantial gains in productivity and innovation. However, a growing concern accompanies this transformative power: the risk of exacerbating social and economic inequalities, particularly along demographic lines such as race. While algorithmic bias in AI systems rightly receives significant attention, it is only one facet of the complex issue of inequitable AI. To truly create an equitable AI landscape, we must delve deeper into the various forces that drive inequality within the realm of artificial intelligence.
The Promises and Perils of AI
AI technologies have ushered in a new era, offering the potential to revolutionise industries and improve the quality of our lives. These technologies are being utilised to automate routine tasks, develop advanced healthcare solutions, and enhance decision-making processes. However, the distribution of AI’s benefits is far from uniform. Instead, it presents a paradox: while AI promises unprecedented advancements, it also holds the potential to worsen existing disparities, especially among different racial and ethnic groups.
Calls for action from business and government leaders to ensure equitable access to AI’s advantages are becoming increasingly urgent. Yet, every passing day seems to reveal new ways in which AI inadvertently creates or exacerbates inequalities, leading to fragmented, reactive solutions or, worse, a lack of response altogether. Addressing AI-driven inequality requires a proactive, holistic approach that goes beyond fixing algorithmic bias.
To make AI more equitable, we must first recognise and understand the three interrelated forces through which AI can perpetuate inequality:
- Technological Forces: Algorithmic Bias:Algorithmic bias, a well-documented issue, occurs when algorithms make decisions that systematically disadvantage certain groups of people. This bias can have dire consequences, especially in critical domains like healthcare, criminal justice, and credit scoring.
For example, a widely used healthcare algorithm was found to significantly underestimate the healthcare needs of Black patients, leading to inadequate care. Algorithmic bias often stems from either underrepresentation of certain groups in the data used to train AI algorithms or from pre-existing societal prejudices embedded in the data itself.
While mitigating algorithmic bias is crucial, it is insufficient for achieving equitable AI outcomes. To comprehend the full scope of inequality within AI, we must also explore how AI shapes the supply and demand for goods and services, contributing to the perpetuation of inequality.
- Supply-Side Forces: Automation and Augmentation:AI’s capacity to automate and augment human labour has the potential to reshape industries by reducing the costs of providing goods and services. Economists like Erik Brynjolfsson and Daniel Rock have extensively studied this phenomenon, identifying that certain jobs are more likely to be automated or augmented by AI than others.
A telling analysis in the United States by the Brookings Institution revealed that Black and Hispanic workers are overrepresented in jobs at a high risk of being automated or significantly altered by AI. This is not due to algorithmic bias but rather because some jobs consist of tasks that are more amenable to automation, creating strategic incentives for businesses to invest in AI technologies. However, because people of colour are often concentrated in these jobs, the automation and augmentation of work through AI can contribute to inequality along demographic lines.
- Demand-Side Forces: Audience Evaluations:The integration of AI into various professions, products, or services can influence how people perceive and value them. For instance, the knowledge that a doctor utilises AI tools for diagnosis and treatment can impact a patient’s decision to seek medical care from that provider. This shift in demand-side dynamics can affect the value of goods and services, resulting in winners and losers. However, the perception of AI-augmented labour varies among individuals, with some embracing it, some expressing scepticism, and others taking a neutral stance.
Understanding these demand-side factors is pivotal because they intersect with biases against marginalised groups. Professionals from dominant groups may receive more trust when AI is involved, while those from traditionally marginalised backgrounds may face scepticism about their expertise. This can exacerbate inequality, especially for professionals from historically disadvantaged backgrounds.
Despite their significance, demand-side factors often receive less attention in the broader discourse on AI and inequality. This perspective is essential for understanding who the winners and losers are in the AI landscape and how these technologies can perpetuate existing disparities, particularly when people’s perceived value of AI intersects with bias against marginalised groups.
For example, professionals from dominant groups typically benefit from assumptions about their expertise, while equally qualified professionals from traditionally marginalised groups may face scepticism about their capabilities. In the context of healthcare, people may be sceptical of doctors relying on AI for diagnosis and treatment, but this distrust may not manifest in the same way for professionals from different backgrounds. Doctors from marginalised backgrounds, who already encounter scepticism from patients, may bear the brunt of this loss of confidence caused by AI. Demand-side evaluations can magnify these disparities, reinforcing inequality in various sectors.
A Comprehensive Framework for Equitable AI
To foster a future of equitable AI, we must address these three interdependent forces comprehensively, recognising their intricate relationships and ripple effects on one another. A deficiency in any one of these forces can destabilise the entire system, creating detrimental feedback loops.
For example, consider a scenario where a doctor chooses not to utilise AI tools to avoid alienating patients, even if the technology could enhance healthcare delivery. This reluctance not only affects the doctor and their practice but also deprives their patients of AI’s potential benefits, such as early detection during cancer screenings. Moreover, if this doctor serves diverse communities, this decision may exacerbate the underrepresentation of those communities in AI training datasets, making AI less attuned to their specific needs and perpetuating a cycle of disparity.
The tripod metaphor aptly illustrates this interconnectedness: a deficiency in one leg directly impacts the stability of the entire structure, affecting angles, perspectives, and ultimately the value AI offers to its users.
To prevent the negative feedback loop described above, we should explore frameworks that help us develop mental models of AI-augmented labour that promote equitable gains. Platforms providing AI-generated products and services should educate consumers about AI augmentation and emphasise that it complements, rather than replaces, human expertise.
While addressing algorithmic biases and the effect of automation is indispensable, these actions alone are insufficient. To usher in an era where AI serves as a force for lifting and equalising, stakeholders from industries, governments, and academia must collaborate through thought partnerships and leadership to devise new strategies that prioritise human-centric and equitable outcomes from AI adoption. Embracing such initiatives will ensure a smoother, more inclusive, and stable transition into our AI-augmented future.